Ants, stock markets, birds? What do they have in common? Each are examples of complex systems. Why are we talking about them in an urban technology newsletter? Because cities are too. Today we look at complexity in cities, and how technology will both add to that complexity and help us make sense of it.
💬 Hello! This is the newsletter of the Urban Technology program at University of Michigan, in which we explore the ways that technology can be harnessed to nurture and improve urban life. If you’re new here, try this 90 second video introduction to our degree program.
Since it’s also college application season, for high school students and the ones who love them, here’s a good way to learn more about our degree program.
🦋 Working Definition for Complexity
While there is no single definition of a complex system, there are some generally agreed upon characteristics:
Heterogeneous: It is a system composed of many distinct elements that are different from one another.
Decentralized: These elements have a degree of autonomy, lacking any central organization or controller. Nevertheless, local patterns and rules naturally emerge to organize interactions between elements in unpredictable ways.
Interdependent: Because of the system’s high degree of connectivity, it is more than the sum of its parts and, as a result, growth, decay, and other effects can appear exponentially in what are called feedback loops.
Dynamic: Because of their autonomy, elements are able to change their behavior based on different circumstances… and they do!
A colony of ants, the biological systems in our bodies, financial markets, a flock of birds in flight, and weather systems are examples of complex systems that are unstable and decentralized, yet include moments or zones of self-organization and follow patterns that arise from the interaction of their constituent parts.
If you’ve spent time in a city, you probably feel this innately. Urban life can be chaotic, but within bounds. Dynamic, but with rhythms. Jane Jacobs used the term “organized complexity” to describe cities as composed of a variety of actors pursuing their own goals, self-organizing in different ways, and whose future state is difficult to predict based on current conditions. It’s one of the things that makes cities so profoundly interesting, and so very hard to work on.
If only there were ways to categorize different types of cities and understand their underlying logic. 🤔 Scholar Michael Batty has been working on exactly that. He’s one of a category of urban scholarship seeking to use complex systems approaches to explain how cities grow, noting generalizable similarities in urban development across geographic size and population, both today and historically; and discover parallels between patterns of urban growth and the occurrence of fractals elsewhere, like so:
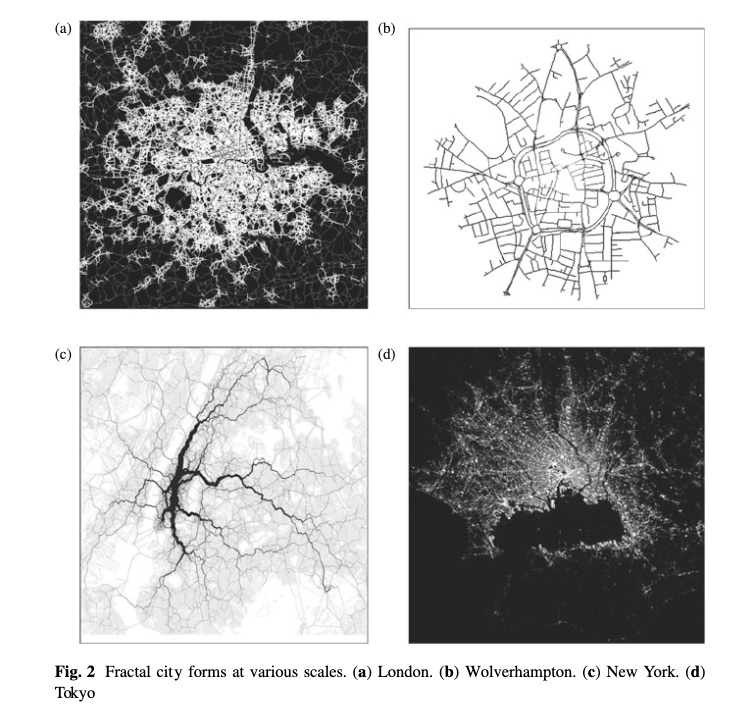
🚕 Hitch a Ride Across Complexity
Cities are composed of a variety of other complex systems. Urban life in Ann Arbor, for instance, is the result of society, nature including birds and insects, and markets for things like real estate (not to mention plenty of other things). Each of these are complex systems in their own right, and each contributes to the complex system of the city. But enough about analogies, let’s look at a very urban example: taxis.
New York is filled with thousands of taxis, each operated by a driver who might be coordinating with a central dispatch unit, but who also has a large degree of autonomy deciding where to go and whom to pick up. From the viewpoint of complexity, it’s a decentralized system in which drivers share common strategies. Taxi drivers want to take the quickest, and generally shortest, route to a rider’s destination, since they want to maximize the number of rides completed and, thus, revenue. Drivers are also competing for customers and will respond to changing demand—if a concert finishes at Madison Square Garden (MSG), it’s in the taxi driver’s interest to head that direction and pick up passengers. That response, in turn, creates new conditions that then affect other drivers: a surge of vehicles headed to MSG will create congestion in the area, meaning that taxis whose route has nothing to do with MSG will be forced to take different routes than they might otherwise. Enough traffic, and that congestions begins to affect buses, cyclists, and pedestrians as well.
Now throw in Lyft, Uber, and other rideshare companies. Many of the same rules of complexity apply, but technology adds other layers: the act of requesting a ride now occurs digitally, and some of the decision-making is now performed by computers, not humans. The conditions to match ride requests to available drivers become more complicated, too, since the formula is based not just on proximity—which car is closest to the requested ride?—but a variety of other factors and preferences.
Did the person request a vehicle with a car seat? Does the vehicle need to be wheelchair-accessible? Did they request a shared vehicle, or a private one? The matchmaking software has its digital hands full, optimizing wait times, available drivers, anticipated routes, etc.—while also updating its decisions based on the latest traffic jam at MSG.
When traditional taxis are busy, it may be harder to catch a ride but the price stays the same. With ride hail the price is generally dynamic and set based on demand, so here we have another aspect of complexity as fluctuations in price certainly also loop back and affect demand.
📊 Managing Complexity
It’s easy enough to analyze supply and demand for something like taxis and come to the conclusion that after prices go up, up, up for a while they eventually revert to the mean. Try to predict peak prices, or the incline of the price slope up or down, however, and these are harder to accomplish. It’s one thing to recognize a system as being complex, but what about discovering the rules and strategies that actors follow within that system? And if you can discover local patterns and adaptations, how how do you find ways to intervene when every action you take within a complex system will cause ripple effects through the system itself?
To take our earlier example: how do you improve traffic in a metropolis like New York City? Or how do you make a more pedestrian-friendly, walkable city? These are problems that are, well, complex. A complex systems approach offers a way to tackle issues that are difficult to define; where there are a variety of stakeholders, all with their own interests; and where any intervention may cause other, unintended effects. Because this approach holds that global behaviors emerge from local interactions, it follows that making a small adjustment at the local level can produce changes in behavior throughout the system, thanks to feedback loops.
Consider the 15-minute city, an idea where residents would be able to meet most of their needs within a short walk (or bike ride) from their home. A traditional approach might pursue this goal from a macro level, deliberately planning neighborhoods and adding (or incentivizing) what we imagine to be an adequate mix of restaurants, businesses, public transportation, and other services within a certain radius of housing. A complex systems approach instead examines local phenomena in aggregate and then identifies patterns that emerge from the data.
A recent study of Barcelona examined location-based data to understand how residents’ movements correlated with different services and amenities, and as a result, the authors were able to offer specific recommendations on what factors could contribute to creating a 15-minute city.
In this case, access to educational facilities, retail, and food amenities were decisive in determining how residents traveled, so planners could theoretically focus on these amenities over others. (Importantly, these factors behaved differently at a neighborhood level compared to citywide trends.)
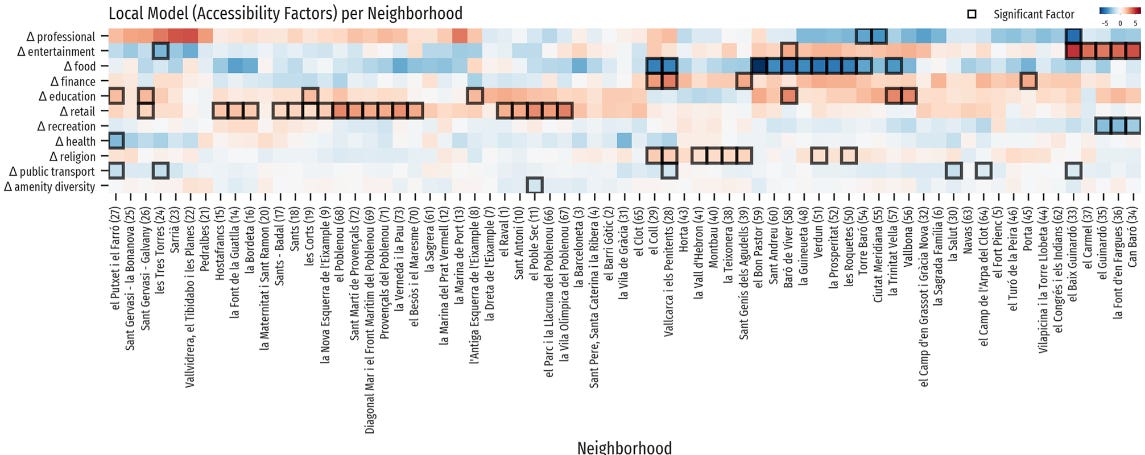
Planners and government officials have long sought tools to understand, and then manage, complex urban phenomena, often by means of simulations to test different policies and approaches. An early example, Cybersyn, was used by the Chilean government to model economic policies in the 1970s, but it didn’t deliver on the promise to serve as an electronic “nervous system” for the country. Cybersyn looked cool, but didn’t work.
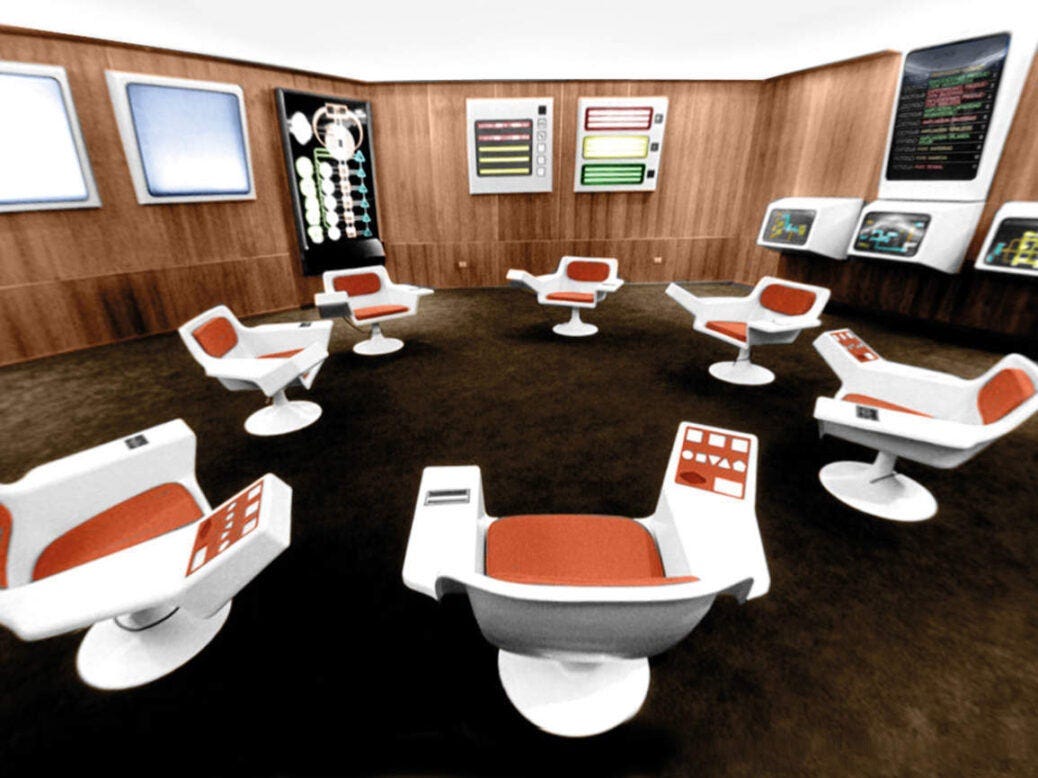
Today, agent-based modeling offers much more sophisticated simulations that can be run many times, and whose variables can be adjusted between iterations, allowing the user of such a model to see the results of different scenarios within minutes. This is an example of applying complex systems theory, since the agent based models are based on the interactions between individual actors, where local interactions can have larger impacts on the entire system.
🎙 Six Questions for Scott Campbell
To further explore these ideas and what they mean for cities, I chatted with Scott Campbell, Associate Professor of Urban Planning here at Taubman College. A lightly-edited transcript of our conversation follows.
CHARLIE KEENAN: For our readers who are less familiar: what is your quick definition of a complex system?
SCOTT CAMPBELL: A simple answer is that the city is a complex system, or society is a complex system. Systems that you can easily set up in a laboratory, that are easily replicated and that have clear instruction manuals are not complex. Have you ever bought a piece of furniture from IKEA? A piece of furniture is not complex—it may be complicated, it may be frustrating, but it's not complex. Often that’s one starting point, because people conflate or confuse the two. A jet engine is complicated because it has many moving parts, but the last thing you want a jet engine to be is complex, because the nature of complexity is that the same starting conditions often lead to rather different outcomes. The last thing you want a jet engine to do is to demonstrate unpredictable behavior.
So, for a complex system, we tend to use the notion that it's a system that learns and adapts. How that learning happens is an interesting question: does it have to involve sentient beings, or can it involve machine learning? Complex systems tend not only to have different agents within it, but agents that are different from one another (that is, heterogeneous). From simple starting conditions unexpected outcomes emerge, which could be crises or instability, but could also be wonderful new things that had not been imagined before. One could apply complexity to notions of urban design, where wonderful new forms of urbanism can emerge, but also disastrous forms.
KEENAN: What’s a good example of a complex system within a city that we can think about, versus fixating on the city itself as complex?
CAMPBELL: That's a great distinction. It's often tempting to say, “a city itself is a complex system,” but often it's hard to deal with the city as an entirety, so instead we can think of the city as a system of systems, whether those are nested or hierarchical or so on. One example would be transportation, or traffic, which is often a popular example of a complex system because it has agents—drivers—who are responding to diverse stimuli; they have goals they adapt by learning where to drive or not to drive, what time to start a trip, or where to turn or not to turn.
You can think about the phenomenon of someone tapping their brakes a little too hard on the freeway, which triggers a chain reaction where, if you're driving and traffic suddenly slows down almost to a crawl, you start waiting to pass an ambulance or tow truck thinking there’s been an accident, but there are none. The whole thing could have just been triggered by someone breaking too aggressively. One can easily experiment with these kinds of traffic scenarios using agent-based modeling software, such as open source NetLogo.
KEENAN: How does the addition of contemporary technology to urban environments affect complex systems?
CAMPBELL: The first thing I think of are Waze and Google Maps and other tools. The nature of complex systems is that agents have the ability to take in new information and then make decisions and change their behavior based on that information—and it’s not just additional information, but new kinds of information they didn't have before, from new kinds of sources.
In the old days, people discussing their commutes around the proverbial water cooler would say, “Oh, you should try this detour.” Now you hear stories about communities in New Jersey having to close some roads because people have discovered through Google Maps or Waze that those roads are really great shortcuts to get on the George Washington bridge. So people have access to types of information they didn't have before, and they have much more rapid, real-time access to it. It just distributes much faster.
So driving is one thing, but then thinking of Uber and Lyft—whole sectors have really changed in a completely unpredictable way. I'm sure there are other examples, but again, that's one of the natures of complexity: when these new technologies are introduced, they are introduced to serve a particular purpose, because you have to convince the venture capitalist or whomever to implement them so they have the intended outcomes, but there are also unintended outcomes that can be both positive and negative.
Think about the poor cab drivers in New York City who paid enormous sums for their medallions and now have to compete with rideshares. Or you mentioned autonomous vehicles. If you live outside New York City, you can take public transit into the city because it’s a pain to drive in the city and parking is so expensive. But if you had an autonomous vehicle, it could just drop you off at the Museum of Modern Art and you wouldn’t have to worry about parking. It’s convenient for you, but you’re then creating unintentional problems with congestion, you’re probably going to discourage public transit, and so on.
KEENAN: How does complex systems offers a unique way of intervening in urban problems and creating solutions to them? If our urban technology or urban planning students study complex systems, what are the tools they would learn to tackle these kinds of issues?
CAMPBELL: One of the mantras I hear from people in complex systems is that traditional predictability doesn't work. We used to do models that were very mechanistic: tell me the starting variables, and I can tell you the outcome. The complex systems people say, it’s not that easy. With one starting condition, you can run the models many times, and you're going to end up with many different answers. Which is a real pain, because one of the tools of the trade in planning is that we not just shape the future, we’re supposed to predict it!
Conventional programming could be predicting demand for housing, population growth, demand on roads, on public services, on and on, over the next 10 to 20 years. That’s what we're supposed to do, and suddenly the complex systems people come along and say, those models don't necessarily work so well.
One of the themes you hear is that to see what actually happens, you need to run the simulation, you can't just calculate from the starting condition 20 years from now. That's where agent-based modeling is a useful tool. You can run different scenarios, and you can speed up the clock—you can have 10 years of urbanization happen in 10 minutes. You can make it an interactive model as well, so that you can run it again and change both starting conditions and interventions, whether that’s introducing a congestion charge if you drive into downtown London or exploring different tools to manage traffic bottlenecks around bridges in the San Francisco Bay Area. Do you increase tolls? Do you allow electric vehicles to go in the free carpool lane? You can test different regulations and see their effects in different scenarios.
One of the hardest things to model is human behavior—we’re fickle, we’re complex—and I think the promise of agent-based modeling is that you can animate agents that aren’t tied to one specific behavior: they can adapt and change behaviors over time. I think that's part of the nature of cities, people are always gaming the system. They're always trying to figure out how to get somewhere faster, to make more money, to get around constraints, to get around rules. Traditional models have a really hard time with that because we make very simplistic assumptions about how people will behave, and people just don't behave that way.
KEENAN: Can you give an example of human behavior in a city that’s especially difficult to model?
CAMPBELL: I haven't seen a lot of work done on this yet, but I worked for a while on a task force for pedestrian safety and access. Trying to protect pedestrians from getting hit is really complicated because pedestrians are fickle, they're complicated, they do stupid things, they stare at their phones, they step out between parked cars mid-block—and drivers are really complicated and distracted, too, so then each side blames the other. In a way you can call that a complex system. You don't want anyone to die for making one mistake, because you have to assume that people make mistakes.
One goal for the Vision Zero idea, where we envision zero fatalities and serious injuries, is to make a system that not only has redundant safety features but also deals with the complexities of drivers, of pedestrians, of the built environment, of lighting, all these things. I don’t know if automated vehicle companies like Tesla and others are adequately anticipating that kind of erratic behavior when they program their cars to look for pedestrians. I think there may be some interesting potential for modeling there.
KEENAN: One last question: what is your favorite city and why?
CAMPBELL: I have many, but if I had to pick one it would be Berlin. I went to Berlin as a 20-year-old exchange student, and I think it was the city that made me fall in love with cities and triggered my interest in urban planning. I’m old enough that I lived in Berlin when it was still divided, so you got to live in one city that at the time had a wall through it, which was tragic and scary, but where else could you have two such radically different political and economic systems? Also, I love Berlin because it wasn't the prettiest city, but it's dynamic and has so much history. I love the fact that people openly debated the connections between architecture, urbanism, history, and politics—if you don’t want to have those discussions, don't live in Berlin, because it would hit you in the face all the time.
Links
🧑🏫 Beautifully not complex: Paris is implementing “school streets” that are closed to traffic. Parking is replaced with greenery. More of this please.
🇫🇷 Speaking of Paris, here’s a map of Parisian buildings by age. Click the boxes in the legend to turn different eras of construction on/off.
🌉 Sticking with mapping, here’s simple problem that (apparently) requires complex solutions: repairing and rebuilding American Bridges. This visualization of US Bridges by material, age, structural system and more might help. h/t Alex Kobald
🚌 Do you really, really, really love buses? Anthony Townsend, who we interviewed last time, sure does and has shared four updates the Bus Data Working Group at Cornell Tech. ⓵ Can Better Data Unlock A Bright Future for Buses? ⓶ The Bigness of Bus Data. ⓷ From Sensing to Sensemaking: Models, Analytics, and Visualizations. ⓸ Towards A Global Bus Observatory.
These weeks: Recruiting, resourcing projections, meeting people, jiggering schedules, jurying the protogrant entries (a highlight), and registering the very first Urban Technology cohort for their first semester of classes that now officially starts in mere weeks. Whoa. 🏃